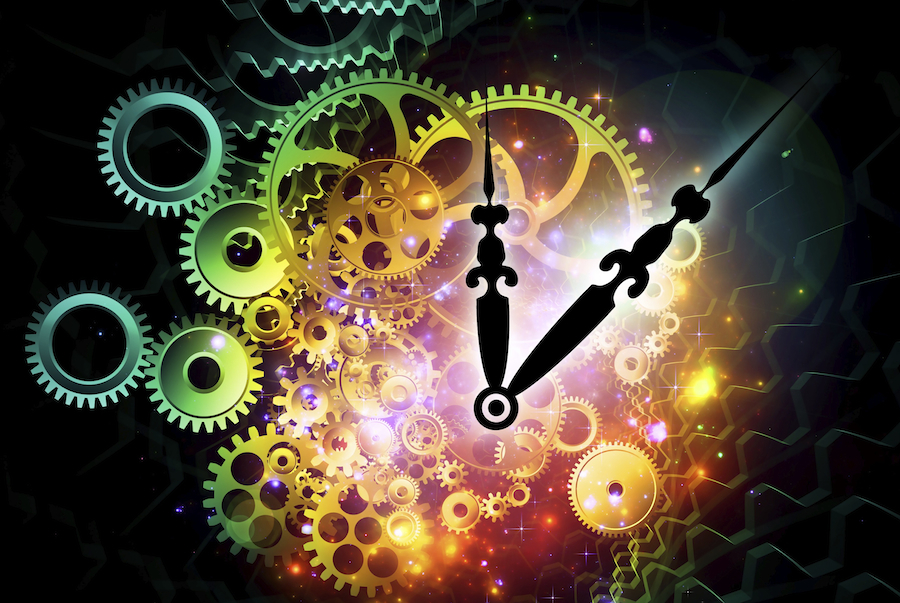
Over the past hundred years, a large body of economics and finance literature has documented monthly seasonality of returns on various assets, such as stocks, bonds, futures, currencies and commodities. More than 150 scientific journal articles, using a variety of data, methods, and time periods, in different markets, have supported the existence of cyclical anomalies.
A major reason why economists are interested in studying seasonal variations is that they are relatively exogenous factors, and hence studying them can help us better understand how markets work. The findings present a potentially serious challenge to classical models of market equilibrium and have stimulated the development of new theories that can account for them.
In recent years there has been a proliferation of empirical studies documenting unexpected or anomalous regularities in security rates of return. These include seasonal regularities related to the time of the day, the day of the week, the time of the month and the turn of the year.
Stock market returns are lowest on average during the fall months when the number of hours of daylight is falling toward its annual minimum. Mean stock returns are then highest as daylight becomes more plentiful in the new year. Numerous researchers report that stocks and bonds of small firms have extremely high January returns, especially during the first five trading days.
A new and interesting strand of research has evolved that investigates the possible impact of weather variables on investor behavior: a strong relation between cloud cover and stock returns, lower stock returns after weekends with daylight savings time changes, lunar phases, temperature.
Small and low priced stocks yield excess returns in the month of January, especially during the first five days. Unusually high returns also occur on the last trading day of December. The turn-of-the-year effect can be interpreted as evidence of a shift in the demand and supply for stocks occurring around the turn of the year. The most widely discussed reason for turn-of-the-year effect in the U.S. is year-end tax selling. Several authors document that the stocks that dropped most in the previous year rebounded most in early January. (Miller, 1990).
Lakonishok and Smidt (1988), from the University of Illinois and Cornell University, examine the potential profits from 1970 to 1981 obtainable by someone who bought the entire volume of stocks traded on the next-to-last day of the year with at least two transactions that day and sold on or after the fifth trading day of January for the stocks in the smallest decile (by trading volume, a criterion closely related to market capitalization) of all stocks on the New York Stock Exchange (NYSE) and the American Stock Exchange (ASE). The calculated return potential was 16.4 percent. This is not an annual rate of return, but a percentage return over about a week.
As one would expect, after the “January effect” was widely publicized, subsequent studies showed that its robustness was declining. The strongest evidence for the effect now comes from smaller countries such as Austria, Denmark, Greece, Brazil, Ireland, Pakistan, Philippines, Hungary, Kuwait, Peru, Portugal, Tunisia, Venezuela, Belgium, and Cyprus.